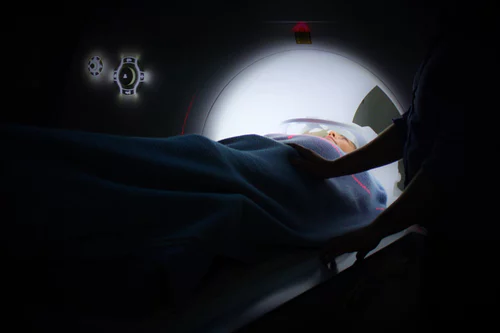
Machine learning model accurately predicts mental illness
Multi-mode, multi-site machine learning examination demonstrated that the predictive model distinguished up to 83% of clinically high-risk mental patients and 70% of introductory depressive patients dependent on 1-year social capacity results.
Past investigations have demonstrated that clinical, neurocognitive, neurophysiological and MRI information can be utilized to anticipate psychosis in individual patients at high danger of clinical results, from Nikolaos Koutsouleris, MD, from the Department of Psychiatry and Psychotherapy at Ludwig-Maximilian University, Germany, and associates. JAMA Psychiatry clarifies that the utilization of machine learning fortifies these discoveries, demonstrating that clinical standard information might be identified with indicators of useful and treatment results in first-episode depression and psychosis.
Koutsouleris and associates express: “Although theoretically proposed, the topic of whether conduct based and MRI-based information can be successfully consolidated in consecutive forecast calculations to streamline predictive power has not been exactly tried.”
This multi-site naturalistic investigation inspected whether clinical, picture based, and joint machine-learning strategies can distinguish indicators of social and job work in patients with high-chance psychosis or early-beginning depression.
The scientists additionally looked at the geographic, cross-demonstrative, and prognostic all inclusiveness of machine learning with human forecasts and surveyed ceaseless results, including clinical and combinatorial machine learning.
The examination included 116 high-hazard individuals with psychological instability and 120 individuals with ongoing discouragement. Koutsouleris and partners distinguished general clinical, imaging, and joint prescient models of social brokenness in patients with expanded danger of psychosis and intermittent dejection.
Utilizing clinical benchmark information, the machine learning prescient model effectively decided the 1-year social capacity result, with an adjusted exactness rate of 76.9% for patients at high hazard and 66.2% for patients with beginning scenes of discouragement. Utilizing basic neuroimaging, the model predicts the forecast of 76.2% of patients with high danger of psychosis and 65% of patients with early dejection. The joint model accurately anticipated a 1-year guess in 82.7% of patients with high-chance status, and 70.3% of patients with ongoing despondency.
The scientists found that the lower work was a trans-sex indicator before entering the investigation. They likewise announced that machine learning performed superior to master conjectures. The outcomes demonstrated that neuroimaging machine learning joined with clinical machine learning expanded the deterministic visualization of patients with high-chance clinical results by 1.9 times, and that of patients with ongoing melancholy expanded by 10.5 times.